This week is all about Design of Experiment aka DOE. DOE is a statistical way for us to plan what we are going to do in experiments, basically planning what will be carried out and taken during an experiment, which will determine the amount of data that will be collected that will help us. This optimization of the data will help us to collate and analyze the data efficiently.
My group is tasked to do 2 case studies and I chose to do Case Study 1.
Factors that are being used in the experiment.
1. Diameter of bowls to contain the corn, 10 cm and 15 cm (Factor A)
2. Microwaving time, 4 minutes and 6 minutes (Factor B)
3. Power setting of microwave, 75% and 100% (Factor C)
8 runs were performed with 100 grams of corn used in every experiment and the measured variable is the amount of “bullets” formed in grams and data collected are shown below:
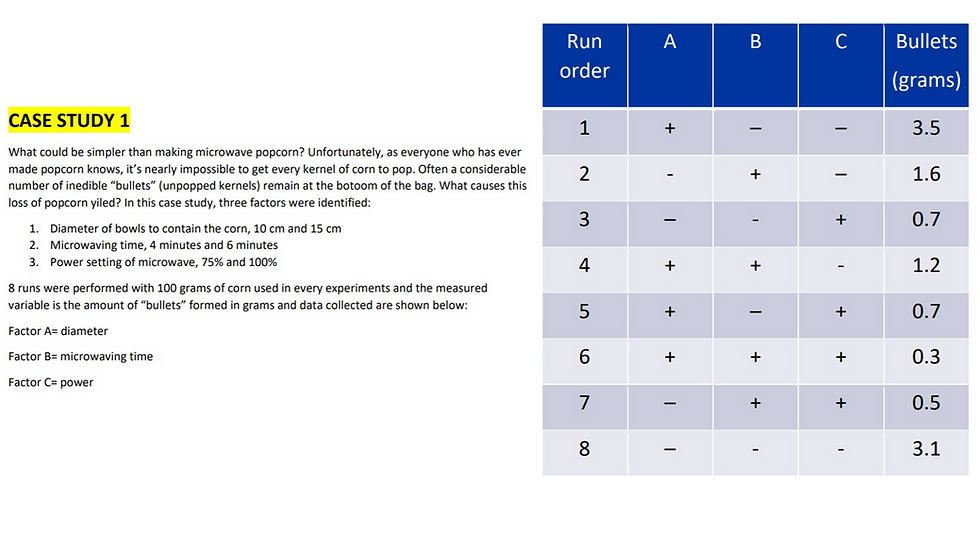
2 forms of data analysis will be carried out, Full Factorial and Fractional Factorial.
I'll start with Full Factorial Design Data Analysis, which will be collecting and using much more data as compared to Fractional Factorial.
Collected Data for Full Factorial Design Data Analysis
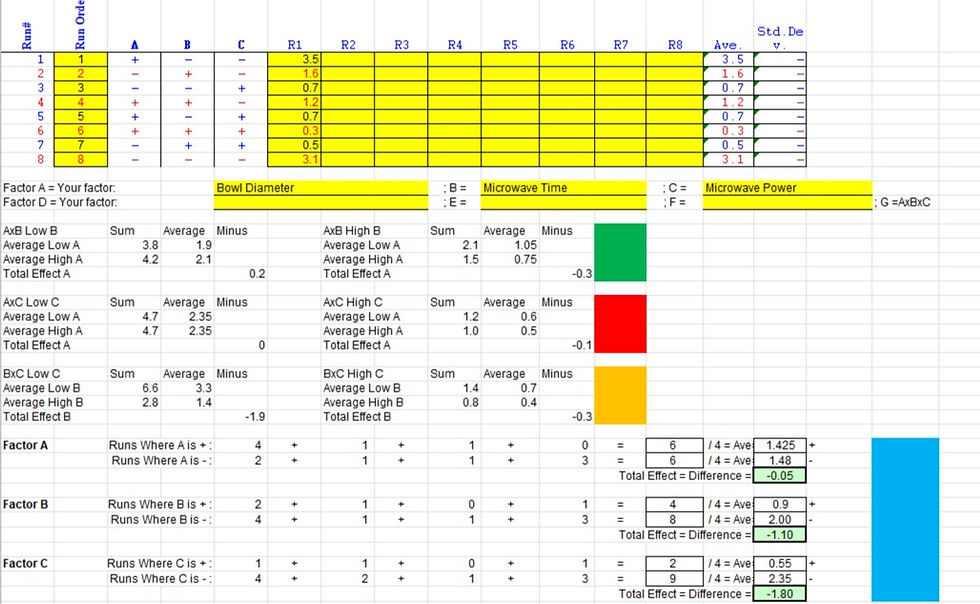
Now, I shall compare and determine each of the interactions, which will be AxB, AxC, and BxC.
AxB Interaction Data and Graph

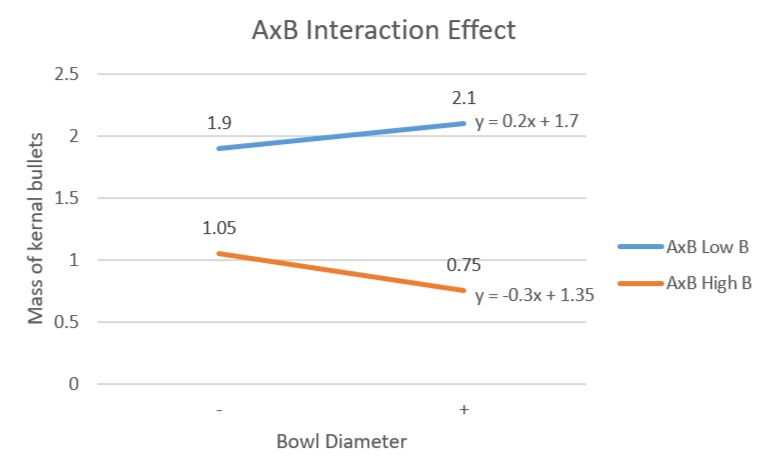
As both lines are shown with little diverging, with gradient difference being very little at 0.1 with each of their gradients at 0.3 to 0.2 respectively, I determine that both the Bowl Diameter and Microwave Time are affecting little or not affecting each other, hence little to no interaction.
AxC Interaction Data and Graph

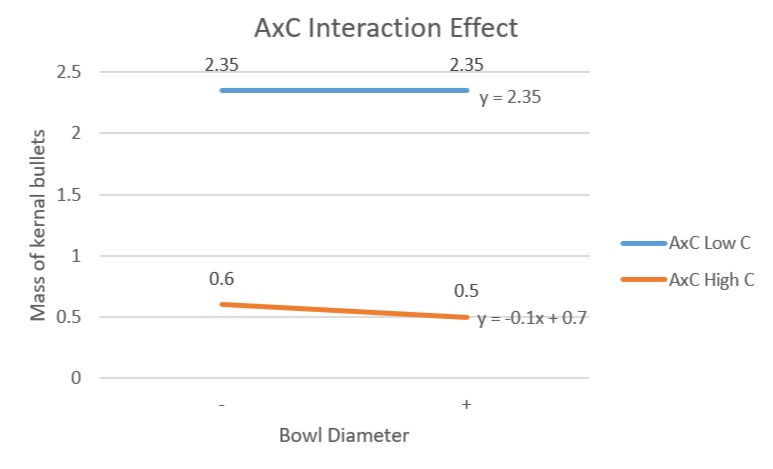
As both lines are shown are almost parallel, with gradients very similar to each other, I determine that both the Bowl Diameter and Microwave Power are not affecting each other, hence no interaction.
BxC Interaction Data and Graph

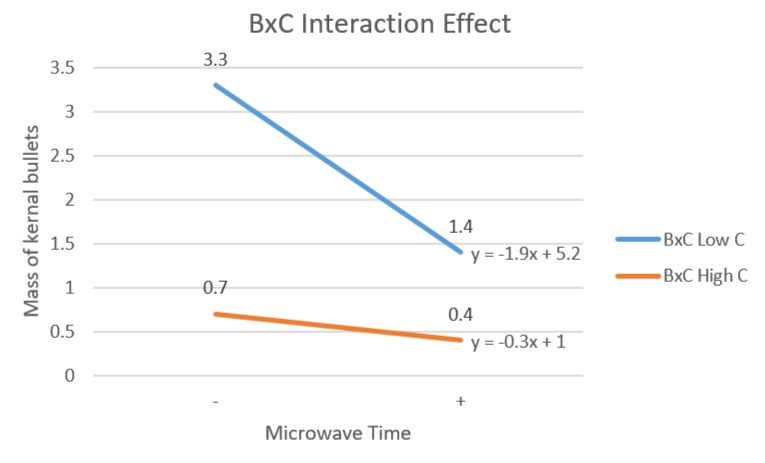
As both lines are shown are at very different gradients, with gradient difference being very large at 1.6 with each of their gradients at 1.9 to 0.3 respectively, I determine that both the Microwave Time and Microwave Power are affecting each other, hence interaction.
Now I shall compare the effects of all individual factors and ranking.
Case Study 1 Full Factorial Data Analysis Graph
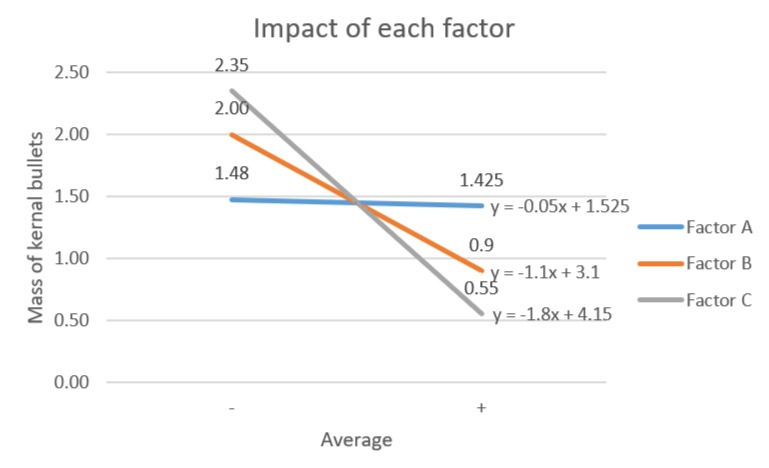
By their ranking from the steepest to the gentlest gradient, C is the first, B is the second, A is the third, with gradients of 1.8, 1.1, 0.05 respectively. This means that Factor C, the Microwave Power has the most impact on the mass of the kernel bullets, while Factor C, the Diameter of the Bowls has the least impact on the mass of the kernel bullets.
Conclusion
In conclusion, I conclude that Factor A, the Diameter of the Bowls has the most impact on the mass of the kernel bullets, while Factor C, the Microwave Power has the least impact on the mass of the kernel bullets. This is largely supported by the gradients shown from the comparison of the effects of all individual factors and the interaction data, which shows the Microwave Power had little to no interaction with the Microwave Time and the Diameter of the Bowl.
Now, I shall do the Fractional Factorial Design Data Analysis, which will be collecting and using lesser more data as compared to Full Factorial. However, this time I will be only comparing the effects of all individual factors and ranking.
Collected Data for Fractional Factorial Design Data Analysis

Case Study 1 Full Factorial Data Analysis Graph
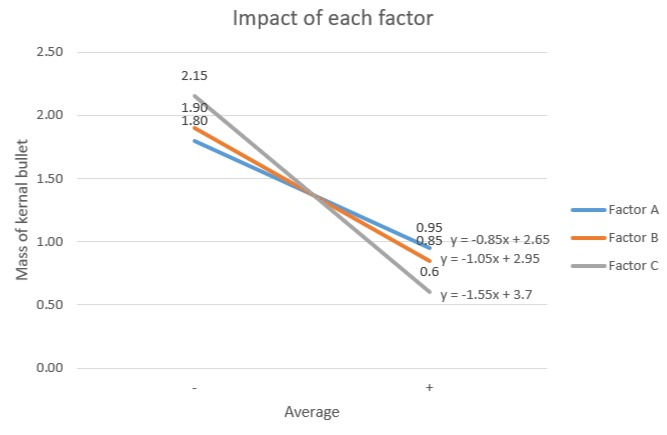
By their ranking from the steepest to the gentlest gradient, C is the first, B is the second, A is the third, with gradients of 1.55, 1.05, 0.85 respectively. This means that Factor C, the Microwave Power has the most impact on the mass of the kernel bullets, while Factor C, the Diameter of the Bowls has the least impact on the mass of the kernel bullets. The trend of ranking of each of the gradients is the same as the ranking in Full Factorial Data Analysis. Also, interaction data cannot be conducted as there is not enough data to show the interaction of each parameter.
Download link
Google link to Excel Sheet of both Full Factorial Data and Fractional Factorial Data
Direct download in Microsoft Excel to Excel Sheet of both Full Factorial Data and Fractional Factorial Data
Reflection:
Overall, I find this practice is tedious as since this is practice, I have to experience taking Full Factorial Data and Fractional Factorial Data, to see which is sets of data is more efficient to analyze This practice is also hella interesting as taking this data really does show me what parameter affect each other and has the most impact, which was able to help me when conducting the experiment and analyzing the data for the practical for the catapult. This would help me in testing the prototype in both CPDD and Capstone Project.
Comentarios